We are working on a manuscript, in which we propose a data-driven distributionally robust optimization approach for addressing the challenges in appointment scheduling problems with random service durations and no-shows. Our manuscript will be posted online soon.
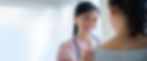
We study a single-server appointment-scheduling problem where the number of appointees and the sequence of their arrivals are fixed, and the appointees have no-show behavior. The service durations and no-shows are stochastic, but the underlying true probability distribution is un- known. With a collection of historical observations, we develop an ambiguity set, which contains the true distribution with a pre-determined statistical confidence level. We then develop a dis- tributioanlly robust optimization model, which minimizes the worst-case total expected cost of appointment waiting, server idleness, and server overtime, by optimizing the scheduled arrival times of the appointees. Under some mild conditions, we reformulate the resulting problem into a tractable convex program, and for the general case, we propose a copositive program- ming reformulation, which motivates a tight semidefinite-programming-based approximation. We validate the effectiveness of our approach on benchmark scheduling instances in the existing literature.